Abstract
INTRODUCTION
Frequent admitters to hospitals are high-cost patients who strain finite healthcare resources. However, the exact risk factors for frequent admissions, which can be used to guide risk stratification and design effective interventions locally, remain unknown. Our study aimed to identify the clinical and sociodemographic risk factors associated with frequent hospital admissions in Singapore.
METHODS
An observational study was conducted using retrospective 2014 data from the administrative database at Singapore General Hospital, Singapore. Variables were identified a priori and included patient demographics, comorbidities, prior healthcare utilisation, and clinical and laboratory variables during the index admission. Multivariate logistic regression analysis was used to identify independent risk factors for frequent admissions.
RESULTS
A total of 16,306 unique patients were analysed and 1,640 (10.1%) patients were classified as frequent admitters. On multivariate logistic regression, 16 variables were independently associated with frequent hospital admissions, including age, cerebrovascular disease, history of malignancy, haemoglobin, serum creatinine, serum albumin, and number of specialist outpatient clinic visits, emergency department visits, admissions preceding index admission and medications dispensed at discharge. Patients staying in public rental housing had a 30% higher risk of being a frequent admitter after adjusting for demographics and clinical conditions.
CONCLUSION
Our study, the first in our knowledge to examine the clinical risk factors for frequent admissions in Singapore, validated the use of public rental housing as a sensitive indicator of area-level socioeconomic status in Singapore. These risk factors can be used to identify high-risk patients in the hospital so that they can receive interventions that reduce readmission risk.
INTRODUCTION
The rising demand for hospital resources from an ageing population and increasing chronic disease burden is putting a strain on the healthcare system and exerting pressure on finite healthcare resources. Patients who are frequently admitted to hospital contribute to bed shortage and also experience significant psychological stress and financial burden.(1) The numerous reasons for frequent admissions include poor health, unresolved medical issues at discharge, poor health literacy and socioeconomic deprivation.(1) Evidence suggests that low socioeconomic status (SES) is associated with readmission in Western health systems,(2-5) and readmission risk prediction models that included socioeconomic indicators have performed better than models without these indicators.(6,7) However, this relationship is less clear in Asian populations due to differences in economic strength and the limited availability of universal social health insurance.(8) Direct out-of-pocket payments may cause the poor to forgo unaffordable hospital treatment.(8)
Singapore is an affluent Asian economy with a gross domestic product of approximately SGD 73,000 and a multiethnic population of 5.6 million people.(9) It has one of the most rapidly ageing populations in Asia, with an increasing chronic disease burden. Healthcare expenditure is expected to triple from SGD 4 billion in 2011 to SGD 12 billion in 2020.(10) The main cost driver of healthcare in Singapore and globally is inpatient cost.(11) In 2010, the 30-day all-cause readmission rate in Singapore was 11.6%, rising to 19.0% among patients aged 65 years and older.(12) This rate is only slightly lower than the 30-day readmission rate in the United States, which was 19.6%.(13) The Ministry of Health (MOH), Singapore, sees the rapidly ageing population and escalating healthcare costs as major concerns. Taking a population-based approach to health, the MOH created six regional health systems (RHSs) in 2011, each responsible for care integration in a specific geographic region. Each RHS is anchored by a tertiary hospital, which is supported by a community hospital providing intermediate and rehabilitation care, with links to primary care and long-term care services in the region. The RHS under SingHealth is the largest healthcare cluster in Singapore and provides care for the south-central part of the country. Our hospital, Singapore General Hospital (SGH), Singapore, is the flagship hospital of the SingHealth RHS and the largest tertiary hospital in Singapore, with 37 clinical specialities and 88,000 inpatient admissions each year.(14) SGH is supported by a community hospital, Bright Vision Hospital, which provides intermediate care to patients requiring inpatient rehabilitation to improve in their activities of daily living. Primary care is provided by the nine public-funded polyclinics under the SingHealth cluster and privately run general practitioners. Community services include day rehabilitation centres, nursing homes, and home medical and nursing services.
Frequent admitters in Singapore (defined by the MOH as patients with three or more inpatient admissions in a year) are high-cost patients with an average cost of nearly SGD 30,000 per patient.(15) Frequent admitters have been found to be older and have the comorbidities of heart failure and stroke.(15) The impact of socioeconomic factors and clinical factors such as high-risk medications and laboratory values remains unknown, although this information would be beneficial in guiding risk stratification and designing effective interventions to improve care delivery for these patients.
The advent of electronic health records allows rich clinical data to be retrieved from electronic data repositories, although individual-level indicators of SES, including individual employment status and monthly household income, are rarely recorded or available in health records. To examine the association between SES and frequent admissions in Singapore, a potential solution could be to use public rental housing as an area-level measure of low SES. In Singapore, home ownership is a key local indicator of SES.(16) In 2014, 5.3% of resident households resided in public rental housing,(17) which the Housing and Development Board (HDB) heavily subsidises for the needy. Households that are eligible for the highly subsidised rates have a low total household gross income of SGD 2,313 per month, compared to the national median household income of SGD 8,290 per month.(18)
Despite the increasing importance of sociodemographic factors for healthcare utilisation, to the best of our knowledge, no study in Singapore has evaluated the impact of low SES on frequent admission risk. The primary objective of this study was to identify the clinical and sociodemographic risk factors associated with frequent hospital admissions in Singapore.
METHODS
This was an observational study using retrospective 2014 data obtained from the administrative database of SGH. It was approved by the SingHealth Centralised Institutional Review Board (CIRB Ref: 2015/2076) with a waiver of patient consent.
To be eligible for inclusion in our study, patients must have had at least one admission to SGH in 2014. Patients who died in 2014, non-residents or patients who had a discharge destination other than home at index discharge were excluded from analysis. Patients without any hospital admission were also excluded, as information regarding their socioeconomic indicators, and clinical and laboratory data were incomplete in the administrative database. We excluded patients who lived in areas where SGH was not the primary hospital, as these patients were likely to be cared for by another RHS. Similarly, patients discharged to long-term residential care facilities were excluded, as they could be located in geographical areas that were not served by the SingHealth RHS and therefore could confound the frequency of subsequent hospital admissions. Ten events per variable was considered as the minimum number required for the calculation of sample size.(19) Therefore, taking into consideration the 30-day all-cause readmission rate of 11.6%(12) and the 37 variables that were evaluated for significance, a minimum sample size of 3,200 was required for our study.
Patient-related data (e.g. demographics, clinical and laboratory data, health services utilisation and mortality) was extracted in a de-identified format from the hospital’s enterprise analytics platform. eHINTS (Electronic Health Intelligence System) is the single enterprise data repository and analytical platform for SingHealth that serves the analysis and reporting needs of business, finance and clinical users. It integrates clinical, operational and financial data from multiple systems and across institutions to provide users with comprehensive information for business decisions. Its analytical tools, ‘self-service’ drill-down capabilities and location analytics enable faster and more efficient reporting and analysis. Data from the Electronic Health Record (EHR) system is integrated to support clinical analytics.
Patient demographics extracted included age, gender, marital status, ethnicity and admission ward class. Variables in our study were chosen a priori and have been shown to affect admission risk, based on the medical literature. As these variables were extracted from the hospital’s EHR and are available to all hospitals in Singapore that use the system, our study findings are potentially generalisable. Our study was novel in that it examined residence in public rental housing (HDB one- and two-room rental flats) as an area-level indicator of SES in Singapore. In our dataset, public rental housing was recoded based on postal codes published on the HDB website.(20) Each housing block in Singapore has a unique postal code.
Clinical data extracted included major diseases listed under the Charlson Comorbidity Index (CCI) and the number of medications dispensed at discharge. Major diseases were identified using International Classification of Diseases, 10th edition (ICD-10), codes in any primary or secondary diagnosis fields dating back to one year preceding the index admission. The CCI was computed for each patient using the Charlson R package. To examine the effect of high-risk clinical conditions, the diagnoses of congestive heart failure (CHF), cerebrovascular disease, chronic obstructive pulmonary disease (COPD) and malignancies were derived using ICD-10 codes in our dataset. Patients with a history of depression were identified through prescriptions of antidepressants they had received, such as lorazepam, mirtazapine, fluvoxamine, escitalopram and fluoxetine. History of using high-risk medications, such as anti-psychotics and warfarin, was also extracted. These were retrieved from data on discharge medications in the EHR. Laboratory values of haemoglobin, white blood cell count, and serum creatinine and albumin were extracted based on the first values recorded at the index admission and used to indicate a patient’s general health condition. Information on prior healthcare utilisation (e.g. number of admissions, and visits to the emergency department and specialist outpatient clinics) in the preceding one year was also retrieved. The outcome measure was frequent admissions in 2014.
Data on health services utilisation, demographics, clinical variables and laboratory variables between frequent admitters and non-frequent admitters was compared. Pearson’s chi-square test and Fisher’s exact test were used for categorical variables, and independent sample t-test was used for continuous variables. Univariate logistic regression analysis was used to investigate the association of demographic characteristics, disease conditions (e.g. CHF, cerebrovascular disease, COPD, presence of malignancies and history of depression) and clinical measures with hospital admission rate among frequent admitters and non-frequent admitters. Factors that were significant in the univariate model were incorporated into the multivariate logistic regression model. All tests of significance used the 95% level (p < 0.05). All analyses were performed using IBM SPSS Statistics version 21.0 for Windows (IBM Corp, Armonk, NY, USA).
RESULTS
A total of 16,306 unique patients fulfilled the inclusion criteria and were analysed. Of these, 1,640 (10.1%) patients were classified as frequent admitters. Patient characteristics are shown in
Table I
Patient characteristics and factors associated with frequent hospital admission.
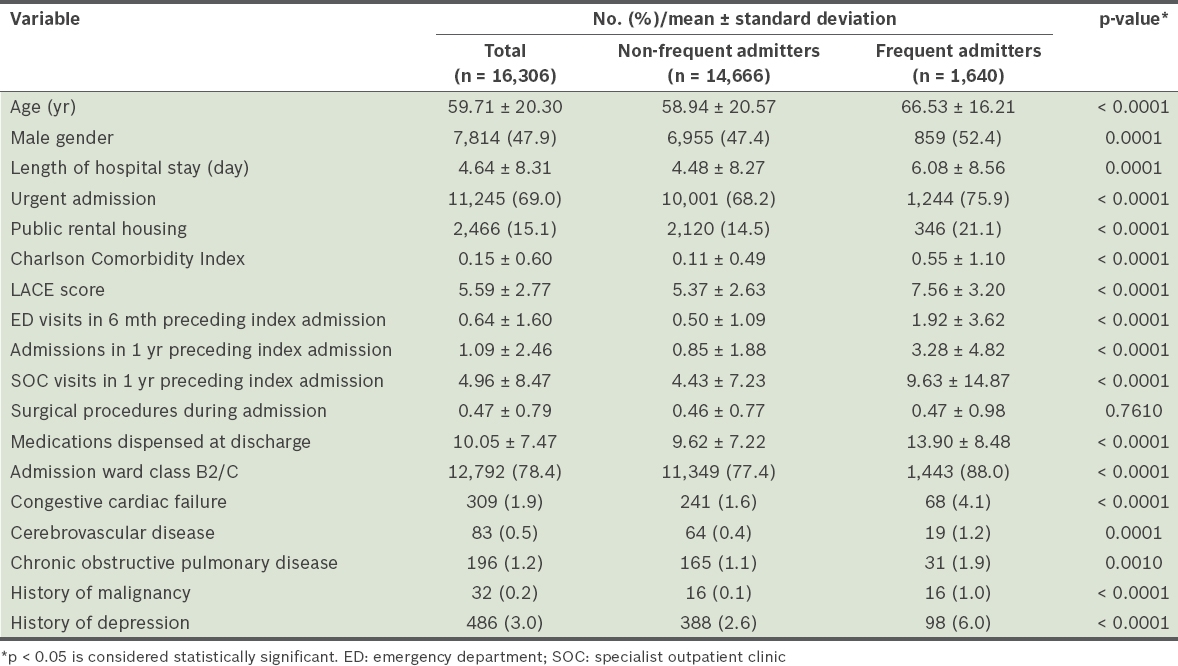
On multivariate logistic regression analysis, 16 variables were found to be independently associated with frequent hospital admissions: male gender; emergent admission; residence in public rental housing; admission to subsidised ward classes; cerebrovascular disease; history of malignancy; haemoglobin level; serum creatinine level; serum albumin level; age; length of hospital stay; LACE score; number of specialist outpatient clinic visits in the preceding one year; number of admissions in the preceding one year; number of emergency department visits in the preceding six months; and number of medications dispensed at discharge (
Table II
Significant variables independently associated with frequent hospital admissions on multivariate logistic regression analysis.
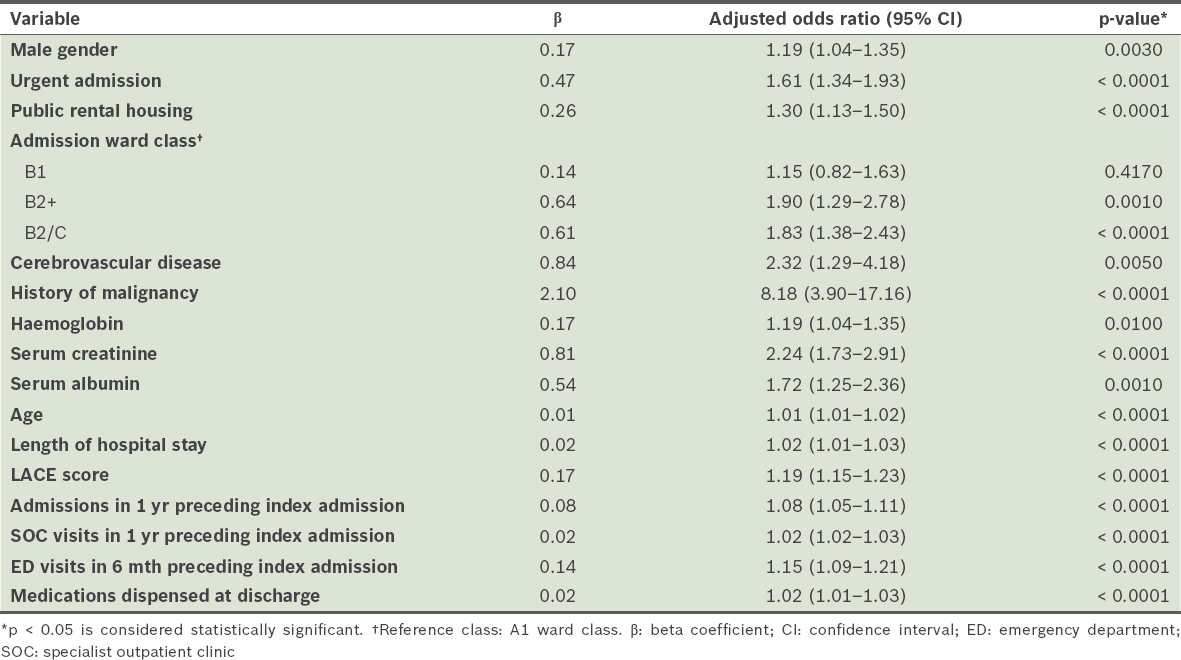
The odds ratio of frequent admitters associated with residence in public rental housing was 1.30 (p < 0.001) after adjusting for demographics and clinical conditions; in other words, patients staying in public rental housing had a 30% higher risk of being a frequent admitter.
DISCUSSION
This study aimed to identify clinical and sociodemographic risk factors associated with frequent hospital admissions in Singapore. Interestingly, we found that patients residing in public rental housing have a higher risk of frequent admission after demographics and clinical conditions were adjusted for, which highlights the importance of social determinants of health.
Possible reasons for poorer outcomes in patients with lower SES include poorer health literacy, the inability of healthcare providers to align their care to the constraints that low SES patients face after discharge, and socioeconomic constraints on patients’ ability to perform recommended behaviours.(19) Locally, Wee et al found that lower-income Singaporeans staying in public rental housing do not view Western-trained physicians as their first choice when seeking primary care,(21) citing cost as a major barrier. The possibility that this delay in seeking appropriate primary care resulted in late disease presentation and inappropriate hospital utilisation warrants further study. Therefore, the responsibility is on the health systems (i.e. RHSs in the Singapore context) to address socioeconomic determinants of health in population health management. Area-level measures of SES, such as public rental housing, can be easily retrieved from hospital electronic medical records and integrated into a real-time prediction model to help clinicians identify patients who are at high risk of frequent admissions. In contrast, while individual-level measures of SES (e.g. individual employment status, being a recipient of financial aid and monthly household income) may be more sensitive indicators, this information needs to be collected prospectively, limiting its practical utility in the busy hospital ward setting. The association of public rental housing with various healthcare utilisation outcomes, such as 30-day readmissions, emergency department attendances or outpatient specialist clinic visits, could be the subject of future studies.
Not surprisingly, debilitating diseases such as cerebrovascular disease and malignancy significantly increase the risk of frequent admission.(22) Laboratory test values, such as haemoglobin, serum creatinine and serum albumin levels, have been associated with avoidable readmissions(23) and 30-day readmission risk.(6,24) Serum creatinine is a clinical marker for renal failure, while haemoglobin and serum albumin levels indicate patients’ general health and frailty.(25) Laboratory values are likely surrogate markers for medical conditions that predispose a patient to frequent admission risk. A surprising finding was the lack of a significant association between CHF, COPD or CCI and frequent admission risk in our study cohort. COPD has been linked to readmissions in the United States; in 2012, the Hospital Readmission Reduction Program (HRRP) required the Centers for Medicare and Medicaid Services (CMS) to reduce payments to hospitals for excess readmissions related to heart failure.(26) In October 2014, the CMS expanded the HRRP to include COPD.(27) The CCI is a measure of comorbidity burden and has prognostic value for 30-day readmission risk.(28) A possible explanation for the absence of a significant association in our study is that these three factors were confounded by other variables in our group, such as prior admissions and emergency department visits in the preceding one year.
While most studies on readmission risk predictive models have focused on 30-day readmission, risk of readmission or death as an outcome measure, very few studies have examined the risk factors for frequent admission risk. However, the median proportion of avoidable 30-day readmissions was found to be only 27% in a study by Van Walraven et al,(29) raising doubts over the concentration of research on 30-day readmissions. Given that frequent admitters are high-cost patients, more attention should be paid to frequent admissions, as early identification and a proactive preventive approach may result in improved patient outcome.
To the best of our knowledge, our study is the first to examine the clinical and sociodemographic risk factors for frequent inpatient admissions in Singapore. Our findings may contribute to creating a future predictive model to identify patients who are at risk of being a frequent admitter, so that they can receive appropriate interventions. This study is also novel in that it validated the use of public rental housing as a sensitive indicator of area-level SES in Singapore.
Our study was not without limitations. Firstly, our dataset did not include patients who used only outpatient or emergency department services in our health system but had no hospital admissions. However, these patients are at low risk and our study aimed to identify risk factors of high-utilising and high-cost frequent admitters. Secondly, as a retrospective study, our database was limited to variables that are routinely collected and updated in the EHR. Other factors that may contribute to frequent admission risk, such as the level of social support, functional limitations and frailty of patients, were not included in this study. Thirdly, we were unable to confirm out-of-hospital deaths or readmissions to health systems other than ours due to cross-utilisation. However, only a minority of patients die at home in Singapore(30) or utilise services from more than one hospital,(14) and such bias was minimised in our study by excluding patients who stayed in geographical locations served by other RHSs. Finally, although our study was conducted at the largest hospital in Singapore and had a large sample size, caution should be exercised in generalising its findings to other health systems without externally validating these findings.
Future studies should consider the significant risk factors in our study when developing a predictive model to identify patients at risk of frequent admissions, particularly the need to adjust for SES using a sensitive indicator, such as public rental housing. The predictive model can be used to identify high-risk patients for early intervention. To tailor interventions that reduce readmission risk, a needs assessment of frequent admitters is required to obtain a more insightful understanding of the social circumstances (e.g. level of social support and individual employment status), health-seeking behaviour, activity levels and functional ability of these patients, as well as hospital and health-system level factors. A short, easy-to-use needs assessment should be developed, and the integration of such data from primary surveys into the EHR could allow accurate regular updates of readmission prediction models.
In conclusion, our study identified clinical risk factors for frequent hospital admissions in Singapore and found that patients with low SES staying in public rental housing were independently associated with being a frequent admitter. These risk factors can be used to identify high-risk patients in the hospital so that they can receive interventions that reduce their readmission risk.
ACKNOWLEDGEMENT
We thank Ms Kanchanadevi Balasubramaniam, Research Coordinator, SingHealth Medicine Academic Clinical Programme, for her assistance with the statistical analysis.