Abstract
INTRODUCTION
We aimed to assess the attitudes and learner needs of radiology residents and faculty radiologists regarding artificial intelligence (AI) and machine learning (ML) in radiology.
METHODS
A web-based questionnaire, designed using SurveyMonkey, was sent out to residents and faculty radiologists in all three radiology residency programmes in Singapore. The questionnaire comprised four sections and aimed to evaluate respondents’ current experience, attempts at self-learning, perceptions of career prospects and expectations of an AI/ML curriculum in their residency programme. Respondents’ anonymity was ensured.
RESULTS
A total of 125 respondents (86 male, 39 female; 70 residents, 55 faculty radiologists) completed the questionnaire. The majority agreed that AI/ML will drastically change radiology practice (88.8%) and makes radiology more exciting (76.0%), and most would still choose to specialise in radiology if given a choice (80.0%). 64.8% viewed themselves as novices in their understanding of AI/ML, 76.0% planned to further advance their AI/ML knowledge and 67.2% were keen to get involved in an AI/ML research project. An overwhelming majority (84.8%) believed that AI/ML knowledge should be taught during residency, and most opined that this was as important as imaging physics and clinical skills/knowledge curricula (80.0% and 72.8%, respectively). More than half thought that their residency programme had not adequately implemented AI/ML teaching (59.2%). In subgroup analyses, male and tech-savvy respondents were more involved in AI/ML activities, leading to better technical understanding.
CONCLUSION
A growing optimism towards radiology undergoing technological transformation and AI/ML implementation has led to a strong demand for an AI/ML curriculum in residency education.
INTRODUCTION
Rapid progress in artificial intelligence (AI) and machine learning (ML) research in recent years, arising from advances in computing infrastructure and deep learning techniques such as convoluted neural networks, promises what Schwab calls, ‘The Fourth Industrial Revolution’.(1) Ushering in the digital age of medicine, radiology received significant attention for revolution due to the potential for computer vision to transform traditional medical image analysis.(2,3) As true clinical application of AI applications continues to grow beyond the computer lab over the last two years, facile fears of machines replacing human radiologists have shifted towards a more sanguine view of AI-augmented radiology practice.(4-8)
In this capricious epoch, many educators have attempted to explore the perceptions of students towards AI in radiology in anticipation of the next phase of radiology training.(9-12) There are also nascent efforts to emphasise an informatics curriculum within residency programmes and the development of AI/ML interest groups within professional bodies to aid continuing education.(13,14)
Radiology residency in Singapore is a five-year programme modelled after American residency programmes and has been accredited by the American Accreditation Council for Graduate Medical Education International (ACGME-I) since year 2011. However, the legacy left behind by the prior structure, which was based on the United Kingdom Royal College of Radiologists (RCR) specialist training programme, required radiology residents to concurrently complete the RCR Fellowship of Royal College of Radiologists (FRCR) examinations as the main qualifying summative assessment.(15,16) The actual training curriculum is a hybrid of both structures – fundamentally based on the ACGME-I structure with emphasis on domains derived from FRCR examination subdivisions: First FRCR examination (Parts 1) assessing imaging physics and anatomy in the junior residency phase (years 1–3); and Final FRCR examination (Parts 2A and 2B) assessing clinical skills and knowledge in the senior residency phase (years 4–5). Year 5 is analogous to the fellowship year in the American residency system.
In the study guide on non-interpretive skills from the American Board of Radiology core and certifying examinations (updated 2019), candidates are required to understand the core basics of imaging informatics pertaining to file standards, reading room environment, scan workflow consideration, data privacy/security and post-processing imaging.(17) In the RCR clinical radiology curriculum (updated 2016), there is also a similar requirement to understand file standards, storage and processing as part of the principles in the medical diagnostic imaging syllabus.(18) Neither explicitly lists AI/ML or the associated data sciences as part of the informatics curriculum.
Learner needs assessment is defined as the process of determining gaps between current and more desirable knowledge, skills and practices.(19) As proposed by Knowles’ theory of adult education, learners need to feel the necessity to learn, and identifying one’s own learning needs is an essential component of self-directed learning.(20) Our study serves as a beginning subjective assessment of individual learner needs pertaining to AI/ML in radiology, with specific goals to lead into future in-depth research studies in order to guide curriculum planning within the next ten years. We aimed to investigate the attitudes and learner needs in the three radiology residency programmes distributed among nine academic acute hospitals in Singapore.
METHODS
We designed an electronic survey using the SurveyMonkey web application (SurveyMonkey, San Mateo, CA, USA). The survey was developed through the review of literature on AI/ML implementation in radiology practice and its influence on education. It underwent several rounds of internal validation and feedback among the project members and sponsors, namely Singapore Radiological Society Imaging Informatics Subsection (RADII) and College of Radiologists under the Academy of Medicine, Singapore. Review waiver was obtained from the lead author’s institutional review board.
The questionnaire consisted of 20 questions, divided into four sections containing five questions each. All questions contained multiple-choice answers, with the use of a 5-point Likert scale (strongly agree, agree, neutral, disagree, strongly disagree), where appropriate.(21) No identifying information was requested.
The first section collected general respondents’ demographic information and included one question (Question 5) on self-perceived tech-savviness, as inspired by Pinto Dos Santos et al’s study on medical students’ attitude toward AI.(11) The second section explored current experience and attempts at self-learning on the subject. Within this section, Question 6 required the respondents to grade their own familiarity with AI/ML in radiology based on the Dreyfus model of adult skill acquisition.(22) Question 7 listed the broad domains of AI/ML applications in radiology beyond automated feature detection as described by Choy et al.(2) The third section probed subjective perception of AI/ML relating to career prospects, similar in focus to prior survey studies.(9,10) The fourth section examined education needs and expectations. Questions 17 and 18 within this section compared the importance of AI/ML training versus imaging physics, and versus clinical skills and knowledge, respectively, which paralleled the focuses of the First (Part 1) and Final (Parts 2A and 2B) FRCR examinations.(23)
The survey was conducted over two weeks (3 December to 17 December 2018). Access to the survey was distributed through email by the respective residency administrative programme executives using a non-serialised Internet link. A reminder email was sent after the first week. The preamble to the survey declared respondent anonymity by design, use of collected data for national education reforms and journal publication. All medical doctors within the residency programmes were invited to participate: residents and core and non-core faculty radiologists.(24) All faculty radiologists have FRCR or equivalent certification. All three programmes (Singapore Health Services, National Healthcare Group, National University Hospital Services) are ACGME-I certified. Participation in this anonymous survey was voluntary, with no monetary incentives.
At the end of the survey period, results were downloaded into a CSV file. Statistical analysis was carried out using IBM SPSS Statistics version 25.0 (IBM Corporation, Armonk, NY, USA). Subgroup analyses for the last three sections were correlated with the demographic characteristics of the following: (a) seniority (resident vs. faculty); (b) gender (female vs. male); and (c) tech-savviness (yes = strongly agree/agree vs. no = neutral/disagree/strongly disagree).
Responses from the last three sections by participants’ demographics were reported as count (percentage). Group comparison was tested by chi-square test for categorical responses and Mann-Whitney test for ordinal rating scale responses. For simplified descriptive statistics, the categories ‘strongly agree’ and ‘agree’ were combined as agreement, while ‘strongly disagree’ and ‘disagree’ were combined as disagreement. A p-value of < 0.05 was taken as statistically significant.
RESULTS
A total of 125 valid responses were gathered over the two-week period using a common collector link disseminated via email, after excluding three incomplete responses. The response rates and demographics of the respondents are shown in
Table I
Demographics and tech-savviness of respondents.
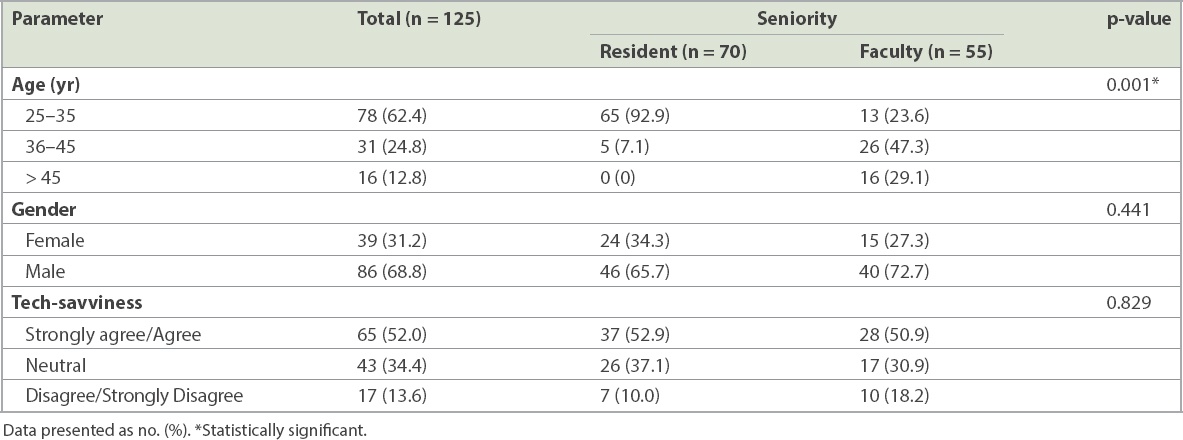
There was a predominance of male (n = 86, 68.8%) compared to female (n = 39, 31.2%) respondents. More than half of the respondents were aged ≤ 35 years (n = 78, 62.4%) and most of the residents (65/70, 92.9%) were in this age group. Out of the 47 respondents aged > 35 years, 42 (89.4%) were faculty radiologists. There was an even mix of respondents from different subspecialty interests, proportional to the estimated prevalence, except for under-representation from cardiothoracic imaging (n = 3, 2.4%;
Fig. 1
Pie chart shows the breakdown of respondents’ subspecialties.
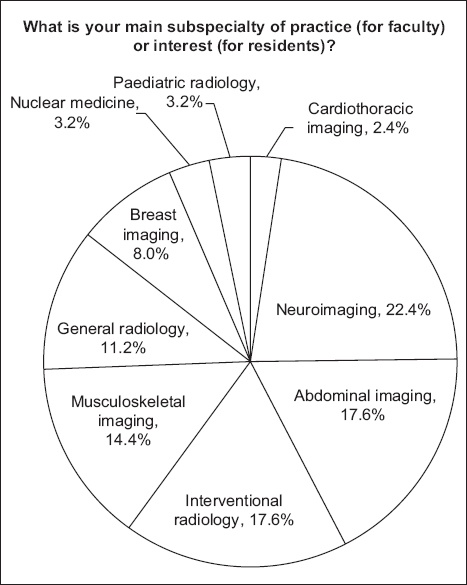
Subgroup analyses in subsequent sections were performed for seniority (resident vs. faculty), gender (male vs. female) and tech-savviness (yes vs. no). Statistically significant associations are specifically mentioned below and summarised in subsequent tables. Since age directly corresponded with seniority (i.e. younger respondents were residents and older respondents were faculty radiologists), subgroup analysis based on age was omitted to remove duplications.
Table II
Respondents’ views on current experience and self-learning of AI/ML.
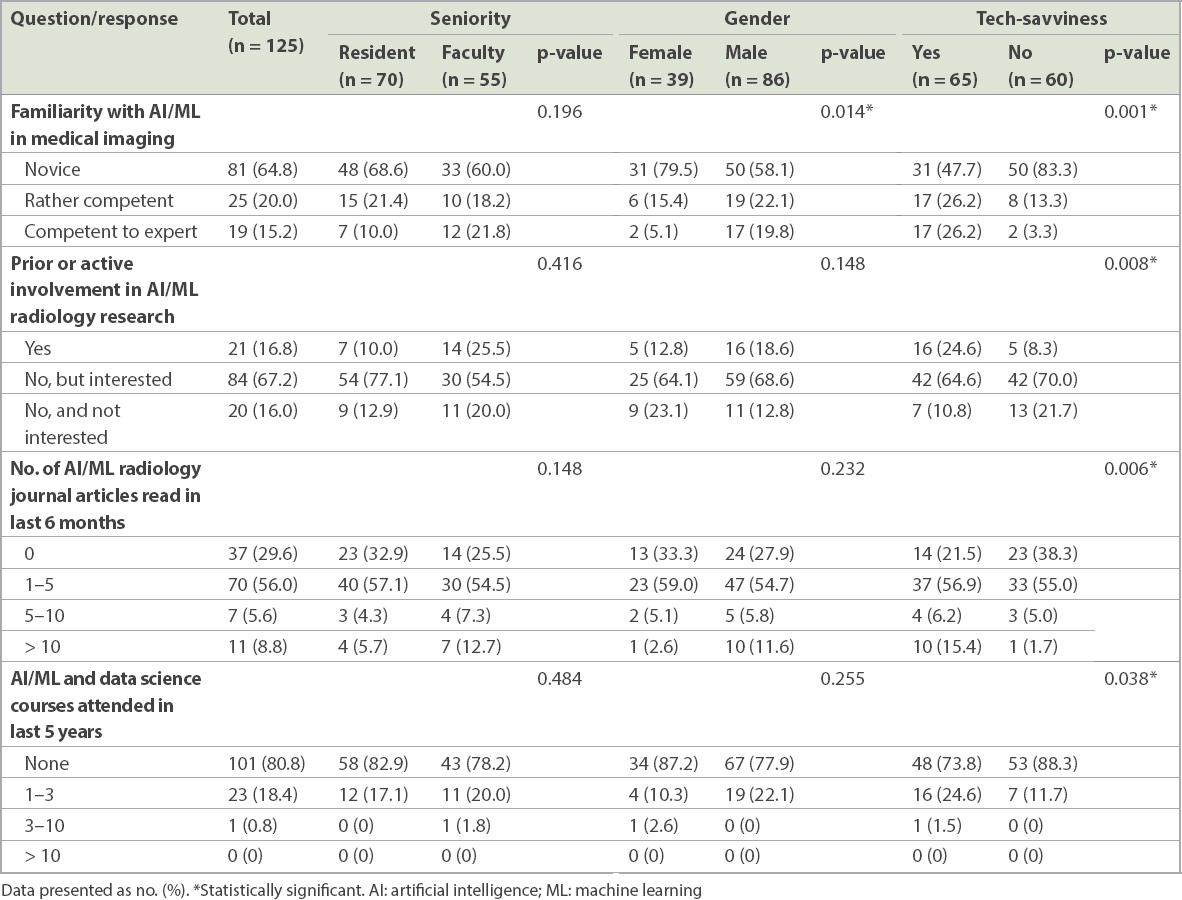
A majority of respondents reported that they had used or were aware of the implementation of some form of AI/ML in their radiology practices (n = 121, 96.8%). This was mainly in the areas of voice recognition transcription and traditional computer-assisted detection, such as in mammography (
Table III
Types of AI/MI applications that participants have used in institutional radiology practices.
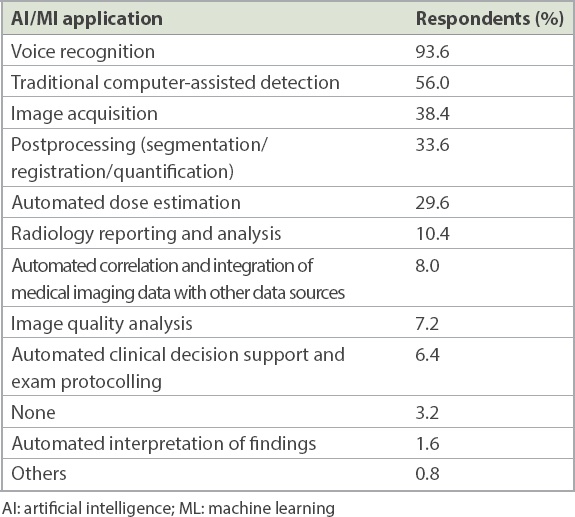
A small majority had read one to five journal articles on AI/ML in radiology in the last six months (n = 70, 56.0%). A higher percentage of those in the tech-savvy group were more likely to have read more than ten articles (10/65, 15.4%), while a higher percentage in the non-tech-savvy group were more likely to not have read any articles at all (23/60, 38.3%). Most had not attended any course on AI/ML or data science in the last five years (n = 101, 80.8%), but those in the tech-savvy group were more likely to have attended at least one course (17/65, 26.2%) compared to those in the non-tech-savvy group (7/60, 11.7%).
The respondents’ views regarding the effects of AI/ML advances on career prospects are shown in
Table IV
Respondents’ views on effects of AI/MI on career prospects.
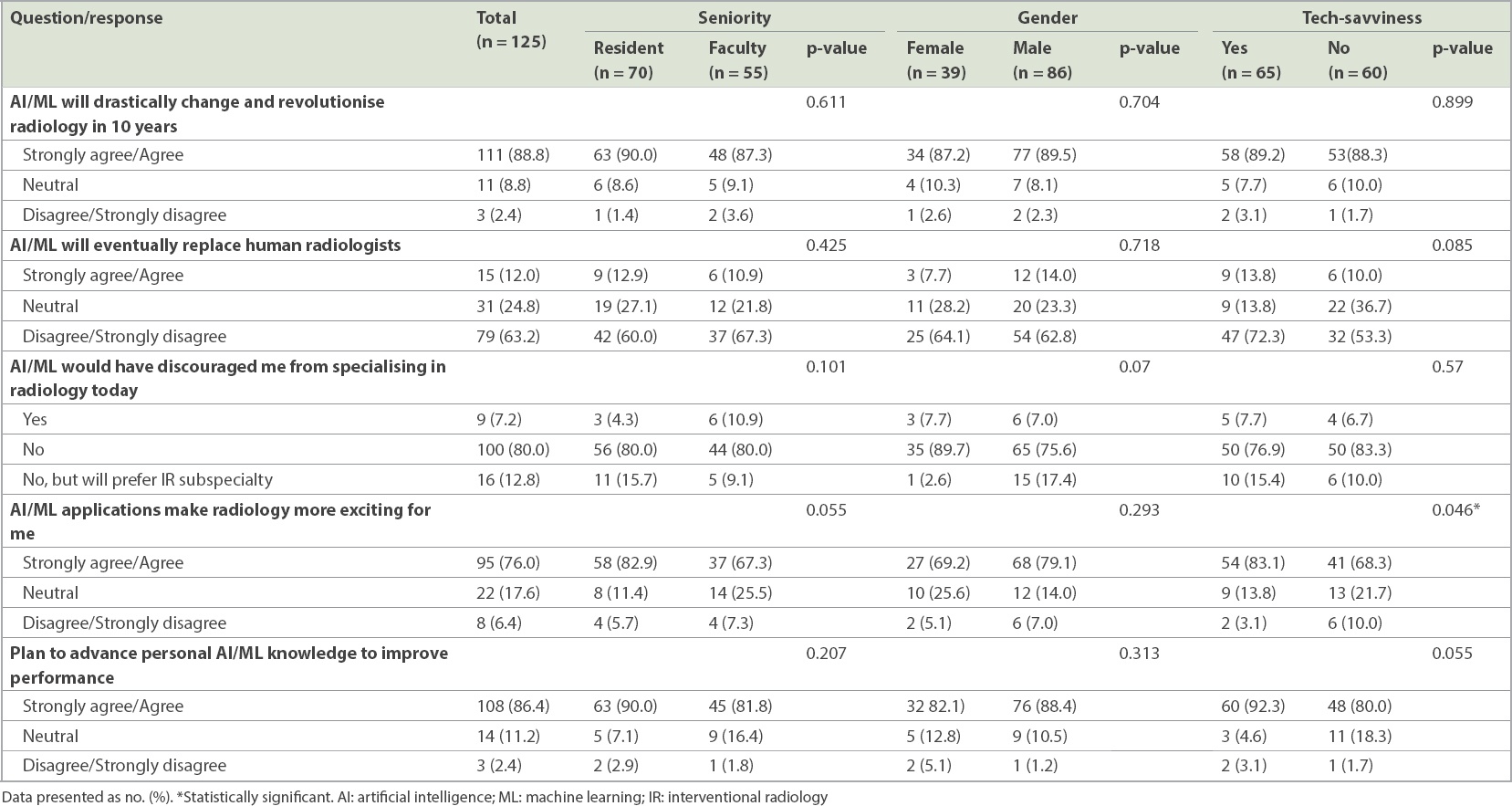
Respondents’ views on education needs and expectations are summarised in
Table V
Respondents’ views on education needs and expectations.
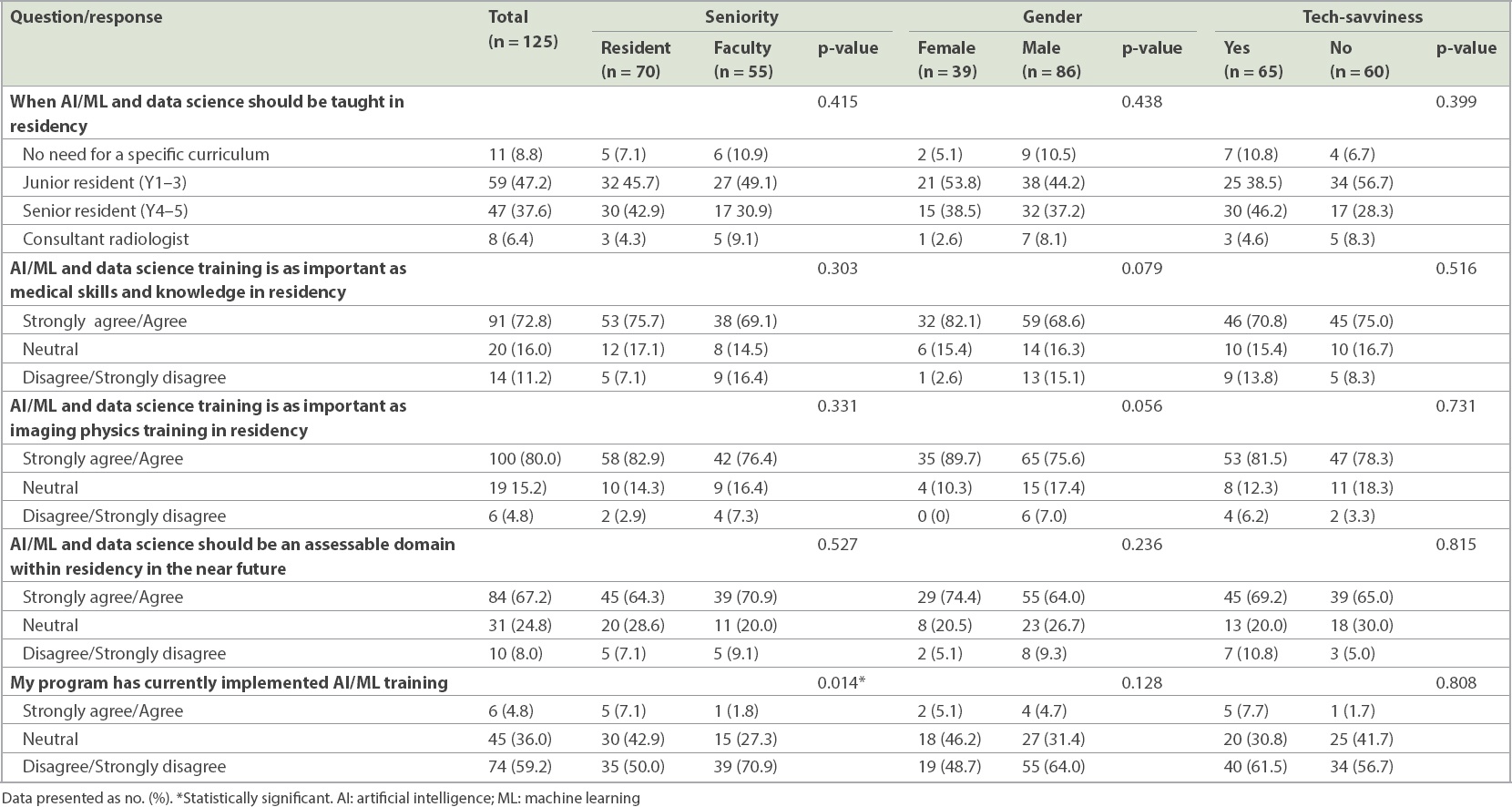
DISCUSSION
AI is the branch of computer science devoted to creating systems to perform tasks that ordinarily require human intelligence. ML is the subfield of AI in which algorithms are trained to perform tasks by learning patterns from data rather than by explicit programming.(25) Use of deep learning algorithms within ML and other related data science techniques is envisaged to be a game changer in radiology, directly pushing evolution of patient care, given the central role of medical imaging.(26)
Our study was designed to gather data that supports upcoming residency curriculum reforms and to serve as a reference point for future comparisons. To the best of our knowledge, this is the first nationwide, multi-programme, multisite survey on the sentiments of residents and radiologists towards AI/ML in radiology with a focus on learner needs.
Sentiments regarding career prospects in radiology were optimistic (
Fig. 2
Graph shows the overall optimism of respondents regarding the impact of AI/ML on career prospects. AI: artificial intelligence; ML: machine learning
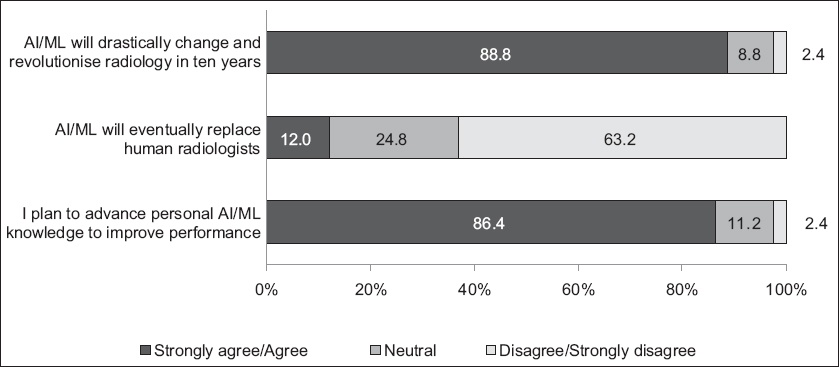
From our results, we opined that there is great demand and untapped potential for developing educational initiatives alongside the radiology AI/ML evolution (
Fig. 3
Graph shows the demand for AI/ML education in radiology residency. AI: artificial intelligence; ML: machine learning
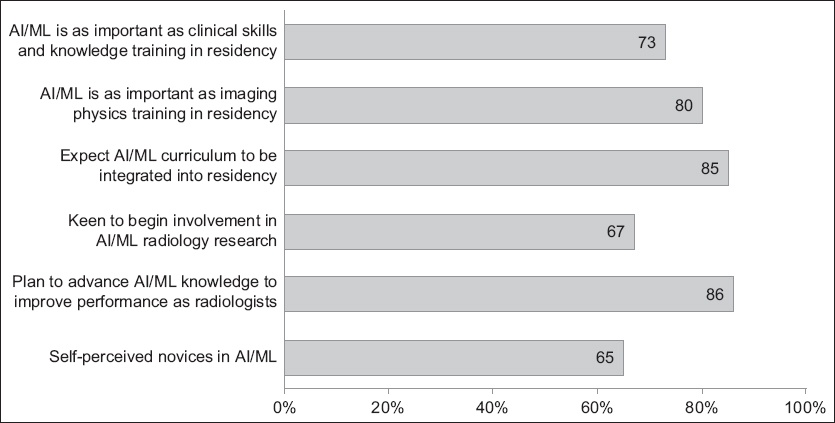
We believe that a radiology AI/ML curriculum should aim for literacy rather than proficiency, allowing radiologists to understand concepts behind algorithms in practice, collaborate with data scientists to uncover clinical usage of AI/ML, and appreciate its limitations, pitfalls and safety issues.(30,32) This is similar to the objectives behind the imaging physics curriculum within the radiology residency programme, which is validated by our results, with 80.0% of our respondents agreeing that AI/ML training is as important as the imaging physics curriculum. Surprisingly, a high 72.8% of respondents also agreed that AI/ML training is as important as the clinical skills and knowledge curriculum and 67.2% believed it should be an accessible domain in the residency programme. Although such expectations may appear overzealous, it highlights the importance of AI/ML training programme to our respondents.
The challenge in any residency programme is maximising training within a limited time-frame through an ever-changing landscape with unlimited content to learn. In the United States, senior residents are sponsored to attend a one-week online National Imaging Informatics Curriculum and Course, which includes basic data sciences and ML concepts as its objectives.(13) In our study, 84.8% of respondents agreed that AI training should begin during residency; a slight majority felt that it should start in junior residency years (47.2%) rather than senior residency years (37.6%). We believe this to be arduous given the breadth of knowledge a junior resident needs to master and the still-evolving nature of AI/ML. A thoughtful AI/ML curriculum, however, might prove to be a deciding factor when trainees are choosing between different residency programmes.
Subgroup analysis using self-perceived tech-savviness gives radiology leadership much to ponder over. Tech-savvy respondents are more likely to feel more competent with AI/ML and more excited about the future of radiology. They are also likely to have read more relevant journal articles, attended courses and participated in AI/ML research. As the prophecy of AI augmented radiology comes to fruition, radiology will continue to attract, and rightfully select, the most technologically inclined doctors to enter the field. We are aware of a self-selecting culture of disruptive innovation percolating through radiology, positioning the discipline as the tech unicorn in the hospital campus. Residency programme directors must continue to encourage technological ardour within their programmes.
On the flipside, we are concerned that the AI/ML evolution in radiology might further discourage women from pursing the specialisation. Radiology specialisation is traditionally less popular with women – a workforce survey study by the American College of Radiology in 2017 revealed that only 21.5% of radiologists in the United States are female.(33) It has been speculated that women may be put off due to the technological inclination of the specialty.(34) In our study, female respondents were more likely to perceive themselves as non-tech-savvy (74.4%) and novices in AI (79.5%). There were no significant correlations based on gender in the rest of the questions, in part due to the small sample size of female respondents (n = 39, 31%). In view of this, residency programmes should be more deliberate in engaging female residents in AI initiatives and understand their unique needs amid traditional cultural biases.
Our results showed little difference between residents and faculty radiologists. In particular, there was no significant difference in self-perceived tech-savviness and willingness to advance knowledge in AI/ML between the two groups, proving that age is no limitation. Compared to residents, more faculty radiologists think that their residency programmes have not adequately implemented AI/ML training in the residency curriculum. This may be due to a lack of awareness of the current programme initiatives or higher expectations of AI/ML training among faculty radiologists.
There are several limitations in our study that are common to voluntary anonymous survey projects. We had good participation from core faculty radiologists (83.7%), fair participation from residents (52.5%), but poor participation from non-core faculty radiologists (11.3%). This led to sample bias, although the nucleus of core faculty and residents within the programmes remained well represented. Also, the final survey questionnaire contained only 20 questions so as to keep within the ideal completion time of 10 minutes, resulting in omission of relevant questions that would have further assessed learner needs from the initial draft.(35) Ideally, we would have sought more information from our respondents about the depth of technical knowledge and the perceived benefits expected from new educational measures. Finally, subjective questions regarding tech-savviness and familiarity with AI/ML are variable in responder interpretation. Objective questions, such as ability to code or assessments on understanding of convoluted neural networks, are easier to interpret but would be at the expense of increased survey length.
In conclusion, our study supports the prevalent positive sentiments towards further technological transformation and AI/ML implementation in radiology. There are pressing needs for inclusion of AI/ML curriculum in radiology education, and residency programmes play an integral role in preparing our radiologists for the next phase. Moving forward, we expect to define objective learner needs in our cohort, introduce formalised curriculum using evidence-based training models and investigate the impact of measures in subsequent follow-up studies.
ACKNOWLEDGEMENTS
We acknowledge the gracious support of the RADII executive and advisory committee, namely Dr Steven Wong Bak Siew, Dr Chan Lai Peng, Dr Andrew Tan, Dr Angeline Poh Choo Choo, Dr Png Meng Ai, Dr Sitoh Yih Yian, Dr Tchoyoson Lim Choie Cheio, Dr Thng Choon Hua, Dr Lionel Cheng Tim-ee and Dr Marielle V Fortier.