Abstract
INTRODUCTION
Unmet psychosocial concerns are associated with emotional distress among cancer patients. This longitudinal study aimed to identify specific psychosocial concern profiles and trajectories of emotional distress, and examine their association among newly diagnosed adult cancer patients across the first year of diagnosis.
METHODS
Adult patients aged 21–64 years were screened to determine their eligibility for this study. Psychosocial concerns and psychological distress were measured using the Problem List and the Distress Thermometer, respectively (n = 221). Latent transition mixture analysis was used to determine specific psychosocial concern profiles and trajectories of emotional distress, and examine associations with adjustments made for demographic and medical variables.
RESULTS
Two classes of psychosocial concerns were identified: low (81%) and high (19%) concerns. Two trajectories of distress were identified: low stable (69%) and high stable (31%) trajectories. Patients in the high concerns class were significantly more likely to demonstrate the high stable trajectory of distress.
CONCLUSION
Our findings highlight the importance of concurrent assessment of multiple psychosocial concerns and screening of emotional distress throughout a cancer patient’s treatment journey. Such assessments can effectively guide interventions to address individual concerns and alleviate emotional distress among newly diagnosed cancer patients.
INTRODUCTION
Cancer diagnosis and treatment is a stressful, life-changing experience for most individuals. Newly diagnosed cancer patients report a plethora of psychosocial concerns including symptom management (e.g. pain, fatigue and nausea),(1) sleep disturbances,(2) body image concerns,(3) financial concerns(4) and fears of mortality.(5) While some patients are able to adjust positively to the illness over time, some may struggle with overwhelming psychosocial concerns, resulting in prolonged suffering and distress.(6) Distress is defined as a spectrum of symptoms ranging from general feelings of low mood and fears to more disabling symptoms of depression and anxiety.(7) Persistent distress is a known risk factor for later depression and anxiety disorders among cancer patients.(8) The prevalence of self-reported depression in newly diagnosed cancer patients is estimated to be between 12.9% and 16.5%, whereas that of anxiety is between 19% and 22.6%.(9) Importantly, there is strong evidence to show that distress, depression and anxiety have adverse effects on cancer patients’ emotional well-being and overall survivorship.(10-12) There is, thus, a need to identify specific profiles of cancer patients who are at higher risk for persistent distress, depression and anxiety disorders, and enhance the clinical utility of current screening assessments in oncology clinics.
There is growing interest, particularly in symptom management research, in identifying specific profiles of cancer patients who are at risk for decreased functional status and poorer quality-of-life outcomes based on presenting physical and psychological symptoms.(13-15) In 2017, Astrup et al conducted latent class analysis (LCA) on 13 out of 32 physical and psychological symptoms measured on the Memorial Symptom Assessment Scale. They identified four distinct symptom profiles among a sample of mixed-cancer patients: higher physical and psychological symptoms were associated with poorer quality of life, younger age, decreased functional status and greater medical comorbidities.(13)
Although identification of patients at risk for poor quality of life based on symptom profiles remains important, clinicians need to understand the importance of psychosocial concerns and their association with distress, depression and anxiety.(6,16,17) There is increased acknowledgement that distress, depression and anxiety are common responses to unmet psychosocial concerns.(16,17) The predominant focus on symptom profiles and general symptoms (e.g. worry) may mask specific, underlying concerns that could be maintaining symptoms of these conditions.(6,16,17) Importantly, it is recommended that interventions should be tailored according to the patient’s concerns.(6,16,17) For example, psychosocial interventions may be provided to address physical concerns (e.g. pain management and sexual-related issues) and psychological concerns (e.g. distressing symptom side effects), while social services and counselling may be provided to address practical concerns (e.g. finances and role adjustments).(17)
To illustrate the limitation of focusing on psychological symptoms, a recent longitudinal study examined the one-year course of distress, depression and anxiety in a large sample of newly diagnosed patients with mixed cancer.(16) It was observed that a significant number of patients experienced distress (51.1%), depression (10.7%) and anxiety (25.0%) at baseline, and that symptoms of distress (29.0%), depression (8.0%) and anxiety (9.0%) decreased across the course of one year.(16) The authors attributed this decrease to better adjustment, which was supported by a significant association between the use of psychosocial resources (e.g. counselling, group support and social services) and a reduction in depression over time.(16) While most patients are able to adjust and cope with cancer, a small group of patients continue to experience persistent symptoms of distress, depression and anxiety over time.(16) The authors suggested that some patients may have more complicated needs that may not be adequately addressed by general psychosocial interventions.(16) However, this study was not able to identify any specific unmet concerns, as it had focused only on examining the course of psychological symptoms.(16) Hence, it was recommended that assessments for psychosocial concerns should be conducted to complement assessments of psychological symptoms and to better connect patients with significant psychosocial concerns and psychological symptoms to the appropriate services.(16)
Oncology clinics in Singapore have incorporated the use of the locally validated Distress Thermometer (DT) to screen for distress among patients and relied on the accompanying Problem List (PL) to identify specific causes of patients’ distress across four psychosocial domains: practical (e.g. finance), personal (e.g. appearance), emotional (e.g. depression) and physical (e.g. pain).(4) Preliminary research in local cancer patients found an 82% prevalence of psychosocial concerns, with an average of 2.8 ± 2.3 problems reflected on the PL (n = 54). Importantly, the overall PL score was significantly correlated with overall distress as measured on the DT, and with depressive and anxiety symptoms on the Hospital Anxiety and Depression Scale. However, the cross-sectional study design and small sample size limited the identification of profiles of psychosocial concerns.
Although identifying psychosocial concern profiles has growing importance in the literature, some studies have cautioned about the utility of such assessment measures.(18) A randomised controlled trial examining the clinical utility of the DT and PL as a form of intervention compared to usual care observed that the DT and PL did not improve distress, quality of life or reduce healthcare costs over 12 months.(18) It is expected that solely relying on the DT and PL will not improve overall well-being and treatment cost-effectiveness; effective cancer management requires both early assessment and intervention that is relevant to and useful for the individual patient. As acknowledged in the study by Hollingworth et al,(18) there is a pressing need to link the results of these screening measures (such as the DT and PL) with individualised supportive care management plans. This is in view of the significant number of patients who were reported to experience persistent depressive (24%) and anxiety (39%) symptoms in the study. However, only a small number (6%–7%) sought help from mental health professionals (e.g. psychologists or counsellors) for these problems. It would be useful to have a better understanding of the reasons for the low uptake of psychological treatment, such as potential stigma or the belief that such services are ineffective. Therefore, the identification of psychosocial concern profiles and their association with distress, depression and anxiety must be linked to appropriate and timely interventions, which may improve the overall effectiveness of cancer management.
To address the gaps identified in the literature, this longitudinal study aims to, first, determine specific psychosocial concern profiles and trajectories of emotional distress based on the PL and DT, respectively, and second, examine their association among newly diagnosed adult cancer patients across the first year of diagnosis.
METHODS
The present study is part of a prospective cohort study (HOPE study) that examined the relationship between psychological resources and psychiatric comorbidities in newly diagnosed cancer patients.(19) The study was approved by the National Healthcare Group Domain Specific Review Board (reference no. 2013/00294), and all participants provided written informed consent.
The methodology and inclusion/exclusion criteria for the HOPE study are detailed elsewhere.(19) In brief, between July 2013 and June 2014, newly diagnosed adult cancer outpatients from the medical, surgical and radiation oncology units of a cancer centre in Singapore were invited to participate in the HOPE study. Inclusion criteria included: (a) age between 21 and 64 years and (b) a new diagnosis of any type of cancer within the past five months. Participants were excluded if they could not communicate in English or Mandarin, were receiving inpatient treatment or had a recurrent cancer within the past two years.
Of the 1,144 patients who were screened, 418 (37%) patients fulfilled these criteria. However, 197 patients declined to participate due to lack of interest or were too ill to participate, resulting in a sample of 221 patients (response rate 53%) (
Fig. 1
Flowchart shows recruitment process and sample selection for the present study.
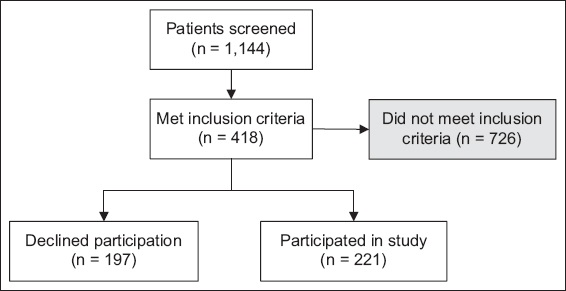
Patients completed self-report measures at baseline that included the following sociodemographic and medical variables: age, gender, ethnicity, education, income, marital status, living arrangement, treatment received or receiving, cancer type and cancer stage.
Psychosocial concerns were measured using a 25-item PL.(20) The PL is a self-report checklist in which patients indicated whether they experienced any of the following problems in the past week: family issues; issues with healthcare staff, finances or bills; lack of information; problems with medication, appearance or self-care; loss of independence; loss of role; sexual or intimacy issues; spiritual issues; anger or irritability; nervousness or anxiety; depression or hopelessness; worry about cancer; issues with memory or concentration, self-esteem or confidence, breathing, eating or weight, or toileting; fatigue or exhaustion; nausea; sleep problems; headaches; and pain. The PL was completed once at baseline.
Psychological distress was measured using the single-item DT, a valid tool for distress screening among local cancer patients.(20,21) Patients self-reported how much distress they had experienced in the past week on an 11-point Likert-type scale (0 = no distress to 10 = extreme distress). The locally validated cut-off score (DT = 5) was used to interpret patients’ trajectories of psychological distress over time. The DT was completed at baseline and at the three-month, six-month and 12-month follow-up.
Latent transition mixture models (LTMMs) contain two latent class variables, each of which was obtained from an LCA and a latent class growth analysis (LCGA).(22) The analysis was conducted in three steps. First, a cross-sectional LCA was conducted on the PL. In particular, the optimal number of latent classes was identified, and their associations with sociodemographic and medical variables were examined. Second, a longitudinal LCGA was conducted on the DT. Lastly, an LTMM was conducted on the latent classes identified from both the LCA and the LCGA. In particular, the latent class variable obtained from the LCGA was regressed on that obtained from the LCA.
LCA is a cross-sectional person-centred approach that identifies smaller homogeneous subpopulations with similar patterns of response to multiple categorical indicators within samples collected from larger heterogeneous populations.(23) In the present analyses, an increasing number of latent classes were fit to the data based on the following statistical indicators: (a) the Akaike information criterion (AIC);(24) (b) the Bayesian information criterion (BIC);(25) (c) the sample-size adjusted Bayesian information criterion (SSA BIC);(26) (d) the Vuong-Lo-Mendell-Rubin Likelihood Ratio Test (VLMR-LRT);(27) (e) the Bootstrapped Likelihood Ratio Test;(28) (f) the approximate Bayes Factor (BF);(25,29) and (g) the approximate correct model probability.(25,30) For each information criterion, better fitting models were indicated by the presence of lower values on each information criterion, and plots were examined to identify elbow joints.(30) For the likelihood ratio tests, the last significant p-value indicated that the addition of any more classes would not provide statistically significant improvements in model fit. The approximate BF indicates the probability that a given class solution is correct relative to one other class solution.(31)
After having identified the optimal number of latent classes in the first of a three-step approach, these classes were related to sociodemographic and medical variables:(32) each patient was classified into one of the latent classes based on modal class assignment, and a model was estimated that accounted for the measurement error due to modal class assignment.
LCGA is a longitudinal person-centred approach that identifies smaller homogeneous subpopulations with similar growth curves within samples collected from larger heterogeneous populations.(30,33) The optimal number of classes was determined based on the abovementioned statistical indicators, and the three-step approach was again used to relate these classes to sociodemographic and medical variables.
After identifying the optimal number of latent classes in both the LCA and the LCGA, the three-step approach was used to fit an LTMM in which the latent class variable from the LCGA is regressed on that from the LCA (a) without controlling for sociodemographic and medical variables, and (b) controlling for sociodemographic and medical variables.(22) Adjustments were made for the following sociodemographic and medical variables: age, gender, ethnicity, education, marital status, type of cancer treatment (chemotherapy, radiotherapy and/or surgery), breast cancer status and cancer stage.
All analyses were conducted in Mplus version 6.12(34) using a full information maximum likelihood approach to handle missing data on indicators of latent classes, which allowed for missing values on these indicators under the ‘missing at random’ assumption. However, because this approach cannot accommodate missing data from patients who were missing on all indicators, a total of 3 (1%) patients were excluded from the LCA and 7 (3%) patients were excluded from the LCGA. The level of statistical significance was set at p-value < 0.05.
Power calculation was conducted using G*Power 3.1.(35) Using the power parameters ([1 − β] = 0.95 and a = 0.05) for two predictors (e.g. two latent class profiles to predict distress trajectories), we required a minimum sample size of n = 107.
RESULTS
The 221 study participants were aged 22–64 (49.21 ± 9.36) years. More than half were female (67%, n = 149) and Chinese (61%, n = 134). The cancer distribution in the sample approximated that of the local incidence profile(36) (
Table I
Baseline sociodemographic and medical characteristics by most likely latent class membership for psychosocial needs and psychological distress.
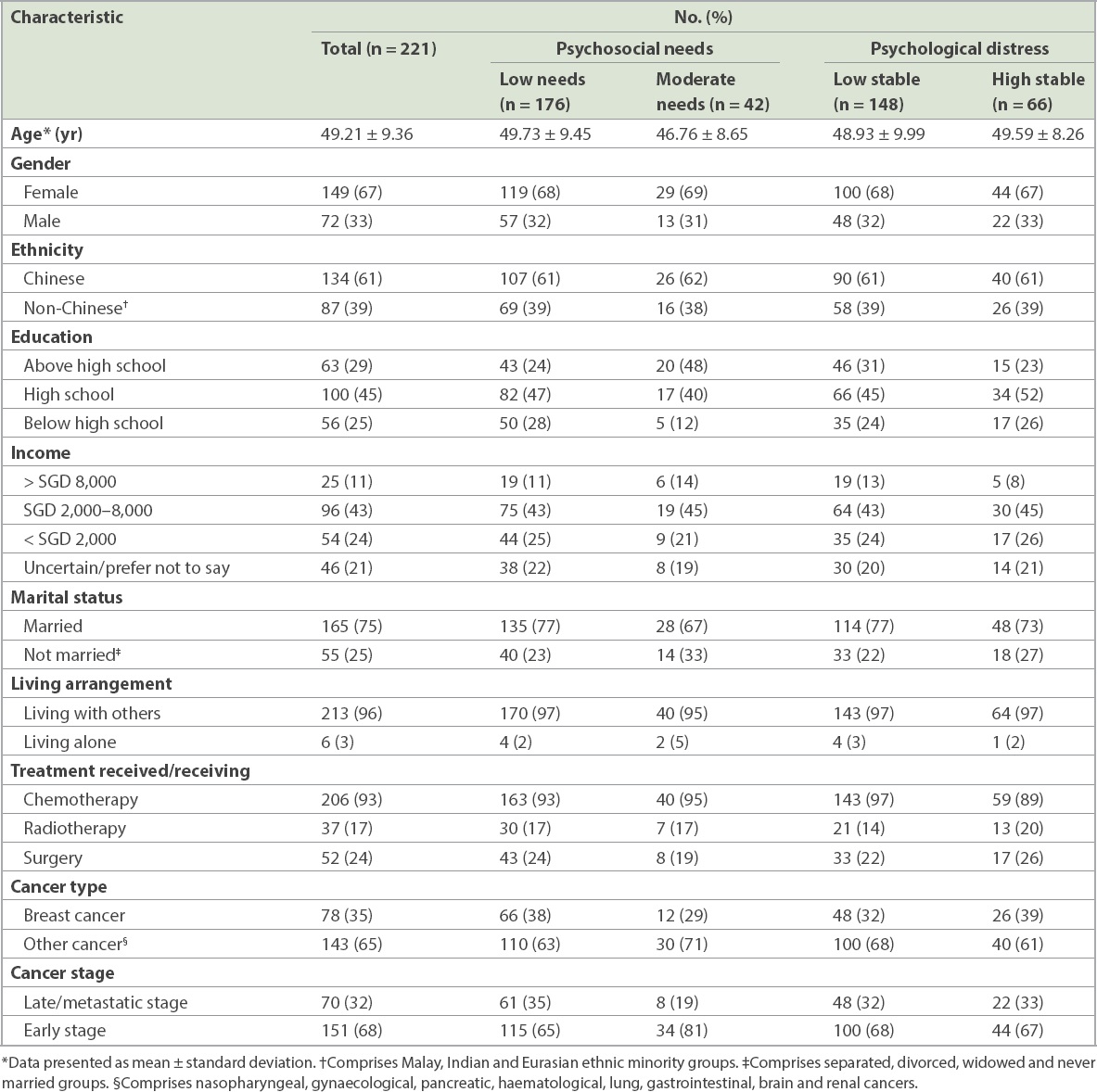
A total of four latent classes of psychosocial concerns were fit to the data in a series of iterative steps; the five-class solution was not well-identified. Among these four latent classes, the two-class solution was determined to be the most optimal based on a combination of statistical indicators: (a) the elbow plots for the AIC, BIC and SSA BIC showed joints at the two-class solution (
Fig. 2
Chart shows the Akaike information criterion (AIC), Bayesian information criterion (BIC) and sample-size adjusted Bayesian information criterion (SSA BIC) at each of the one-class to four-class solutions. The horizontal axis depicts the class solution, and the vertical axis depicts the value on each information criterion.
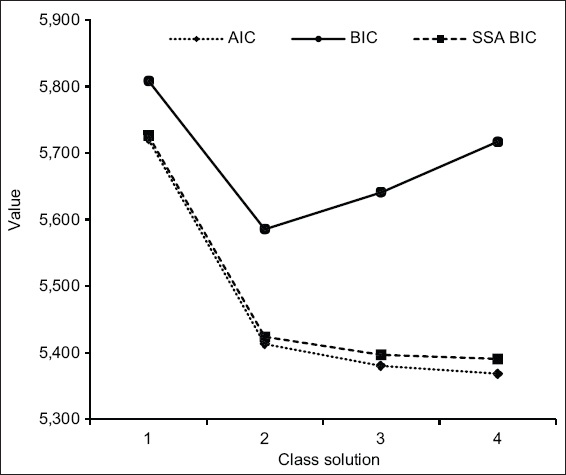
Fig. 3
Chart shows latent classes of psychosocial concerns, with each latent class being defined by a pattern of probabilities across all 25 items on the problem list. The error bars represent 95% confidence intervals, and the dotted line at item probability = 0.5 represents chance.
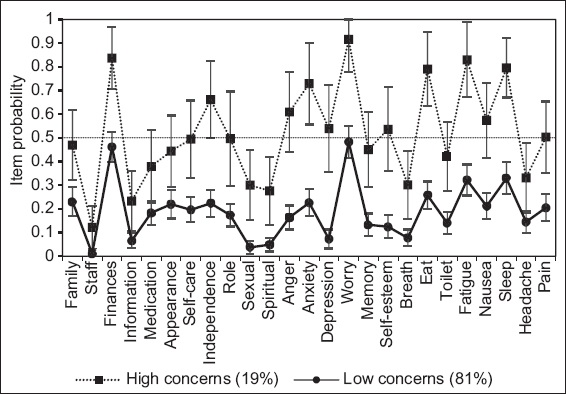
The first class, which comprised 176 (81%) patients, was characterised by low probabilities of endorsing most items on the PL, with the exceptions of finances/bills and worry about cancer, which were endorsed at a rate not significantly different from chance. This class was, thus, termed the low concerns class. The second class, which comprised 42 (19%) patients, was characterised by low-to-moderate probabilities of endorsing most items on the PL, with the exceptions of finances/bills, nervousness/anxiety, worry about cancer, eating/weight, fatigue/exhaustion and sleep problems, which were endorsed at a rate significantly higher than chance. This class was, thus, termed the moderate concerns class.
In general, most sociodemographic and medical variables were not significantly associated with the latent classes (p ≥ 0.063 for all), with the exceptions of age (B = −0.35, standard error [SE] = 0.02, p = 0.045) and education (B = 0.87, SE = 0.29, p = 0.003). In particular, patients who were younger or had received higher levels of education were significantly more likely to demonstrate moderate concerns as compared to low concerns.
A total of six latent classes of distress were fit to the data in a series of iterative steps; the seven-class solution was not well-identified. Among these six latent classes, the two-class solution was determined to be the most optimal based on a combination of statistical indicators: (a) the elbow plots for the AIC, BIC and SSA BIC showed joints at the two-class solution (
Fig. 4
Chart shows the Akaike information criterion (AIC), Bayesian information criterion (BIC) and sample-size adjusted Bayesian information criterion (SSA BIC) at each of the one-class to six-class solutions. The horizontal axis depicts the class solution, and the vertical axis depicts the value on each information criterion.
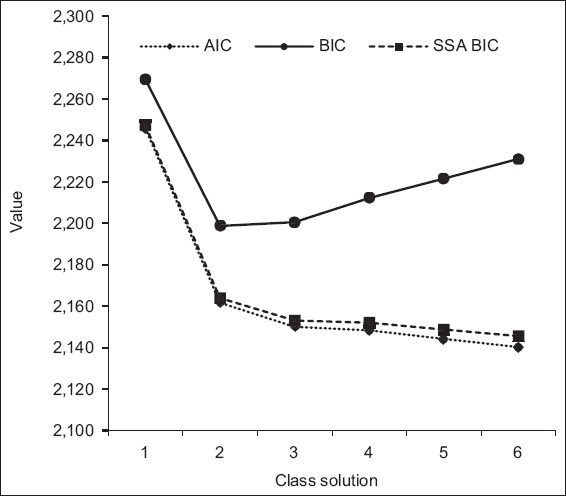
Fig. 5
Chart shows trajectories of distress, with each trajectory being defined by a pattern of means across all four assessment points. The error bars represent 95% confidence intervals, and the dotted line at distress = 5 represents the cut-off score.
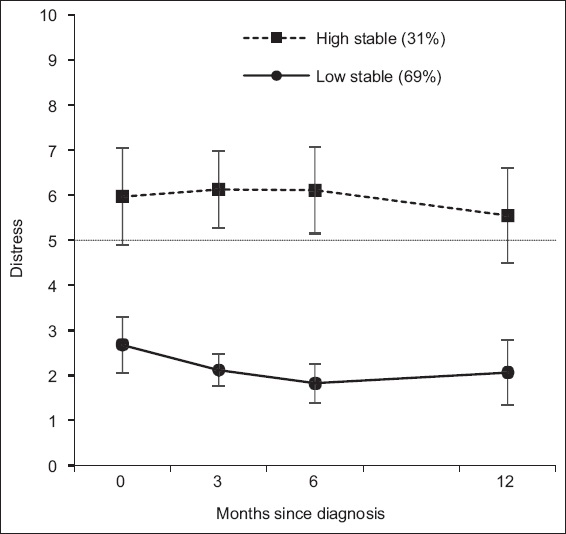
The first class, which comprised 148 (69%) patients, was characterised by low levels of distress immediately following a cancer diagnosis that remained stable across the following 12 months. This class was, thus, termed the low stable trajectory. The second class, which comprised 66 (31%) patients, was characterised by high levels of distress immediately following a cancer diagnosis that remained stable across the following 12 months. This class was, thus, termed the high stable trajectory (
A significant association was observed between psychosocial concerns and distress. In particular, patients with high psychosocial concerns were significantly more likely to demonstrate the high stable trajectory of distress (B = 2.87, SE = 0.77, p < 0.001). This association remained significant even after controlling for all sociodemographic and medical variables (B = 3.82, SE = 1.22, p = 0.002).
DISCUSSION
Extending from previous research on psychosocial concerns among cancer patients, the present study identified two profiles (high and low concerns) of psychosocial concerns among newly diagnosed cancer patients. Our finding is consistent with previous research showing that patients who were at risk for emotional distress were more likely to endorse concerns related to finances, anxiety, worry and sleep on the PL.(37) Other distressing concerns related to fatigue and eating are also well documented in the literature, although past findings were based on different psychosocial concern measures.(1,38) As the majority of patients in the present sample were undergoing chemotherapy, the most frequently reported physical concerns within the high concern profile may be associated with physical side effects due to treatment.(2) Oncologists should clarify these concerns with patients during routine screening so that appropriate pharmacological treatment and advice can be provided.
The present study also observed that both age and education were significantly associated with psychosocial concern profiles. Specifically, patients who were either younger or more educated were more likely to be classified into the high psychosocial concern profile. Our finding corroborates well with previous observations in the literature that younger patients experienced greater emotional distress(37) and higher symptom burden.(12) Younger patients may shoulder more financial and family responsibilities, and the need for aggressive treatment may result in greater concerns over their ability to continue performing these roles adequately. While the association between education status and greater psychosocial concerns remains unclear in the literature, it is plausible that those with higher education status may have greater awareness of the implications of their diagnosis and treatment, resulting in greater psychosocial concerns.
The present study identified two distinct trajectories of emotional distress: low stable (69%) and high stable (31%) trajectories. Previous longitudinal studies have reported inconsistent findings on the overall changes in emotional distress among cancer patients over time.(16,39,40) This variability may be due to differences in cancer types, assessment measures and study duration. However, longitudinal studies examining the trajectories of emotional distress in cancer patients have reported that while the majority of patients adjust positively over time, there is a smaller group of patients who continue to experience persistent emotional distress.(41,42) While the present study did not observe a trajectory of decreasing emotional distress, our findings on persistent emotional distress suggest that there could be important factors maintaining emotional distress, which warrant further examination.
Accordingly, further analyses revealed that patients who were classified into the high concerns profile were more likely to demonstrate the high stable emotional distress trajectory. This may suggest that high levels of unaddressed practical, personal, emotional and physical concerns may be maintaining emotional distress. Hence, identifying a high psychosocial concern profile is essential for subsequent clinical management. Importantly, although patients who endorse high concerns may not experience increasing emotional distress, the experience of persistent distress may worsen over time and increase the risk of depression and anxiety, with severe psychosocial implications.(9,42)
The present study has limitations such as the small sample size and gender skew. Second, no known studies have identified psychosocial concern profiles among newly diagnosed mixed-cancer patients, and direct comparisons are not possible. Further studies are required to replicate the identified psychosocial concern profiles and examine their association with emotional distress trajectories. Third, the present study measured psychosocial concerns and emotional distress based on self-report, which may be subject to response bias. Thus, future studies could incorporate structured clinical interviews to corroborate information obtained on the DT and PL. Fourth, the present study was not able to obtain information pertaining to emotional distress, depression and anxiety prior to diagnosis, although these may have an influence on the level of emotional distress and psychosocial concerns that are reported after diagnosis. Finally, the present study did not collect information regarding any form of psychological intervention received by patients over one year. Accordingly, caution is needed to interpret our findings on distress, which may be influenced by potential psychological interventions.
The findings underscore that it is important for clinicians to identify psychosocial concern profiles and address patient-perceived problems early on during the cancer journey. Furthermore, with an increasing ageing population in Asia, the difficulties faced by older individuals with a cancer diagnosis should be specifically studied. Their experiences may involve different psychosocial concerns with greater impact on their quality of life. In summary, care management for cancer patients should include a detailed assessment of multiple concerns and concurrent screening of emotional distress throughout their treatment journey. This will ensure that interventions and support are better targeted at individual concerns to alleviate emotional distress.
ACKNOWLEDGEMENTS
The HOPE Study, on which this study is based, was funded by the National University of Singapore Start-Up Grants R-177-000-039-133 and R-177-000-039-733 to Mahendran R. The funding body had no involvement in the study design, collection, analysis or interpretation of data, writing of the manuscript, and the decision to submit the manuscript for publication.